Exploring the Importance of Data Annotation Tools and Platforms
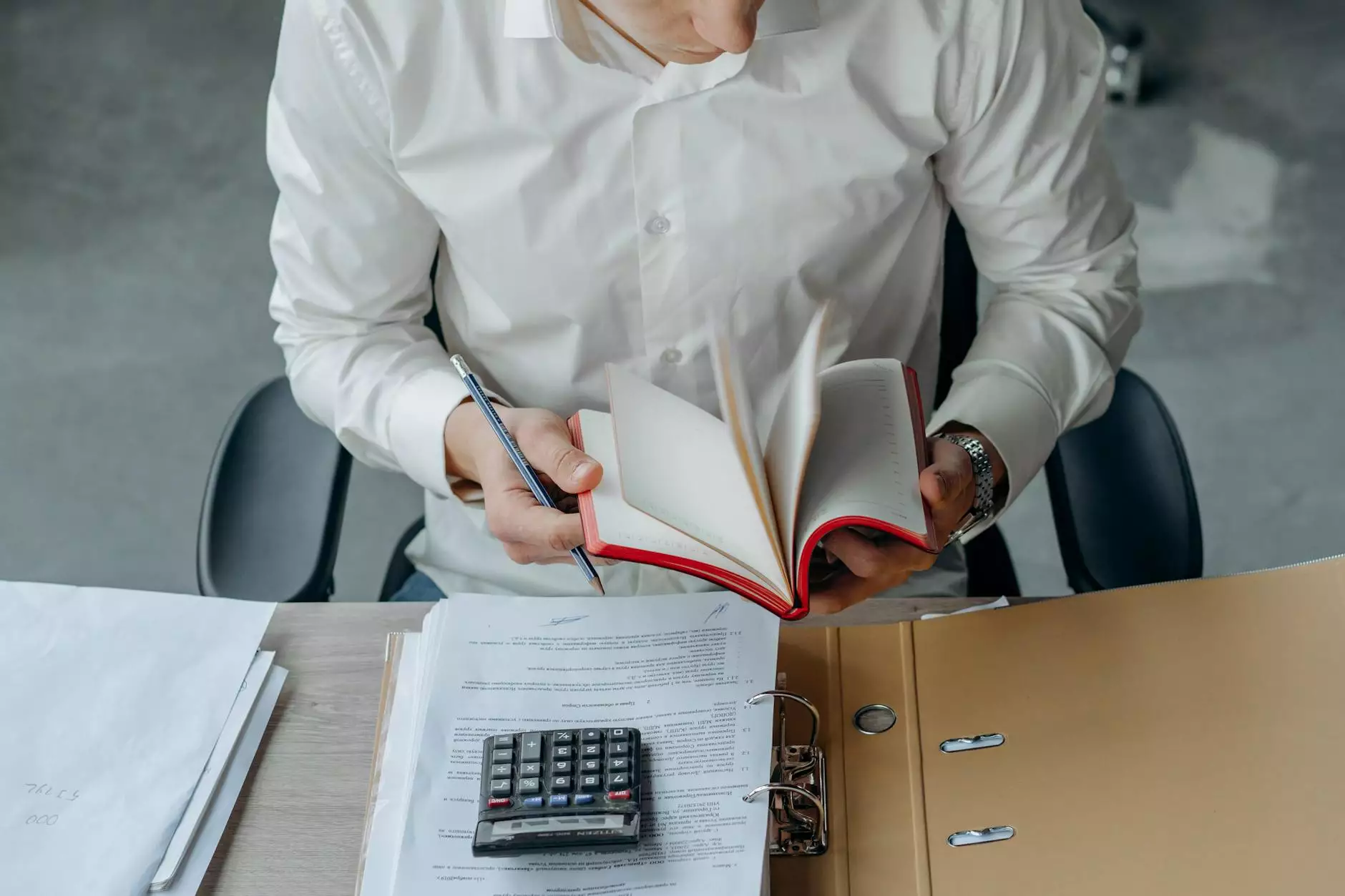
In today’s digital world, where the amount of data generated is growing exponentially, efficient data annotation tools and data annotation platforms are more crucial than ever. These tools are the bedrock of artificial intelligence (AI) and machine learning (ML) systems. Their primary role is to enhance the accuracy of machine learning models by labeling the data that these models use for learning.
Understanding Data Annotation
Data annotation refers to the process of labeling data for analysis. In the context of AI, this means turning raw data into a format that machine learning algorithms can understand and process. For example:
- Text data: Sentiment analysis requires labeling text with sentiment scores.
- Image data: Object detection involves identifying various objects in an image.
- Audio data: Speech recognition systems rely on annotated audio data to learn vernacular and dialect.
Why Are Data Annotation Tools Essential?
Data annotation tools are vital for several reasons:
- Improved Accuracy: Well-annotated data leads to more accurate models.
- Efficiency: Automated tools significantly decrease the time needed for manual annotation.
- Scalability: As businesses grow, so do their data needs. Scalable annotation platforms can accommodate vast datasets.
Types of Data Annotation Tools
There are various data annotation tools available in the market, each designed for specific tasks. Here are some popular types:
1. Image Annotation Tools
These tools are specifically designed for labeling images. They typically provide functionalities such as bounding boxes, polygon tagging, and keypoint annotation. Popular choices include:
- Labelbox
- SuperAnnotate
- CVAT (Computer Vision Annotation Tool)
2. Text Annotation Tools
Text annotation tools are essential for natural language processing tasks. They facilitate the labeling of text data for various applications such as sentiment analysis and named entity recognition. Tools include:
- Prodigy
- DataTurks
- LightTag
3. Audio Annotation Tools
These tools support the labeling of audio data, crucial for developing speech recognition systems. Some of the commonly used audio annotation tools are:
- Audoctave
- Praat
- Audacity
The Keylabs Data Annotation Platform
One of the leading platforms in the data annotation realm is Keylabs. This platform stands out due to its user-friendly interface and extensive functionalities. Let's explore some of the standout features of Keylabs:
1. Comprehensive Annotation Capabilities
Keylabs provides a range of annotation tools tailored for different data types, including image annotation, text annotation, and video annotation. This versatility allows users to manage all their annotation needs under one roof.
2. Collaboration Features
In a world where teamwork is essential, Keylabs facilitates seamless collaboration between team members. Multiple users can work on the same project simultaneously, enabling real-time updates and communication.
3. Quality Assurance Mechanisms
The platform incorporates robust quality assurance processes to ensure the integrity and accuracy of annotated data. This includes review cycles where high-quality standards are maintained.
4. AI-Powered Annotation
Keylabs also leverages AI technology to automate parts of the annotation process, drastically reducing the time required to label large datasets while maintaining high accuracy levels.
Best Practices for Data Annotation
To maximize the effectiveness of data annotation, consider these best practices:
- Define Clear Guidelines: Precise annotation guidelines help annotators understand expectations and reduce errors.
- Regular Training: Continuous training sessions for annotators ensure they are well-equipped with the latest tools and techniques.
- Implement a Feedback Loop: Regular feedback helps annotators improve and adapt to changing requirements.
- Utilize Quality Checks: Establish review processes to catch errors before finalizing datasets.
Challenges in Data Annotation
Despite its importance, data annotation comes with challenges:
- Scalability: As data volumes increase, maintaining quality can be difficult.
- Time-Consuming: Manual annotation can be slow and labor-intensive.
- Subjectivity: Different annotators may interpret guidelines in varied ways, leading to inconsistencies.
The Future of Data Annotation
The future of data annotation will likely be characterized by:
- Increased Automation: AI and machine learning will continue to play larger roles in automating annotation tasks.
- Enhanced Tools: New features in annotation tools will improve efficiency, making it easier to annotate complex data types.
- Integration with AI Workflows: Data annotation will be more closely integrated into AI development pipelines, streamlining the ML lifecycle.
Conclusion
In conclusion, data annotation tools and platforms are indispensable in the realm of AI and machine learning. Investing in robust tools like Keylabs can significantly enhance your project outcomes by ensuring high-quality, labeled datasets. As the demand for accurate AI models continues to rise, so too will the importance of efficient data annotation practices.
https://keylabs.ai/